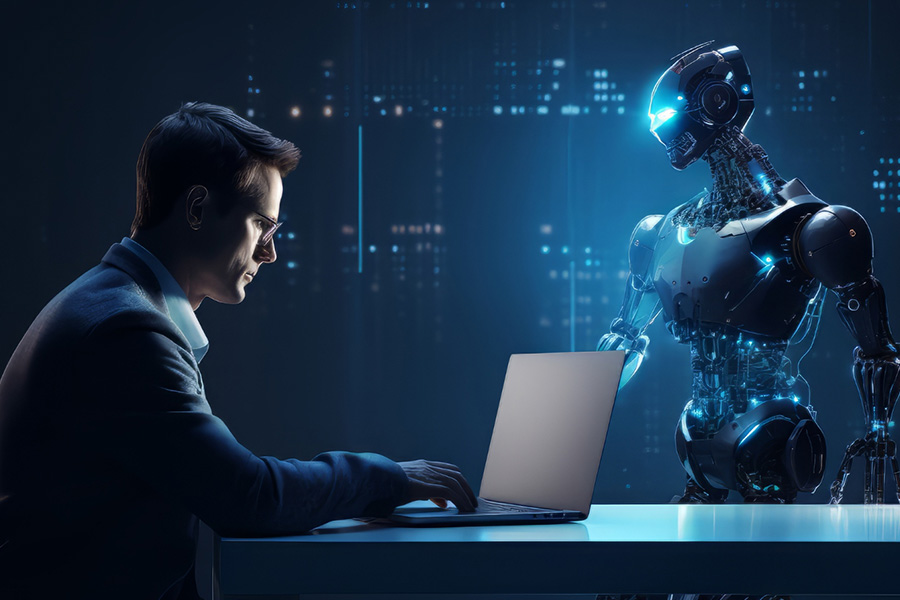
This research developed an ensemble-based contextual classifier for academic papers, combining machine learning and deep learning, to accurately categorize articles and suggest credible publication venues. The system effectively classifies papers into 40 categories and recommends up to 10 potential publication sources, helping researchers streamline their decision-making process when choosing where to publish.
Authors
Abdullah Al Kafi, Department of Computer Science and Engineering, Daffodil International University, Dhaka, Bangladesh
Sumit Kumar Banshal, Department of Computer Science and Engineering, Alliance University, Bangalore, India
Nishat Sultana, Department of Computer Science, William and Mary, Willamsburg, USA
Vedika Gupta, Jindal Global Business School, O.P. Jindal Global University, Sonipat, Haryana, India
Summary
This research aims to develop an automated contextual classifier for scholarly papers by utilizing established algorithms and understanding the information retention of different parts of a scholarly article, such as the Abstract, Article Title, and Keywords. It also seeks to recommend a contextual classifier-based recommender system to help academics identify credible sources. Scholarly articles from various study fields often use similar terms in their titles and keywords. However, finding a publication venue can be challenging for researchers at the beginning of a scientific inquiry. Thus, it is crucial to classify information based on its context, especially when abstracts, keywords, and titles receive equal attention.
Materials and Methods
An ensembled model was developed and trained using 114K instances from 38 classes of the Web of Science (WoS) dataset and 40 classes of the Dimensions dataset. The ensemble approach incorporated both machine learning and deep learning algorithms to build a diverse classifier. The model was evaluated by testing it with an 80:20 train-test split to assess performance. The classifier was further integrated into a recommender system designed to suggest probable publication sources based on given article information.
Results
The ensemble classification approach demonstrated superior performance with faster inference and efficient training time. The balanced training model, tested on 114K instances, effectively categorized scholarly articles into one of 40 categories. The recommender system was capable of recommending up to 10 probable publication sources based on the article’s Title, Keywords, and Abstract. Models utilizing abstractions yielded the best results and provided a better understanding of the context in every iteration of the experiment.
Conclusion
This study successfully developed an ensemble-based contextual classifier for academic papers, which can also function as a recommender system. The system aids researchers in choosing the most appropriate sources to publish by categorizing articles into 40 categories and suggesting credible publication venues. This approach simplifies the decision-making process for academics, enabling them to identify relevant publications and suitable sources for their work more efficiently.
Published in: Journal of Scientometric Research
To read the full article, please click here.