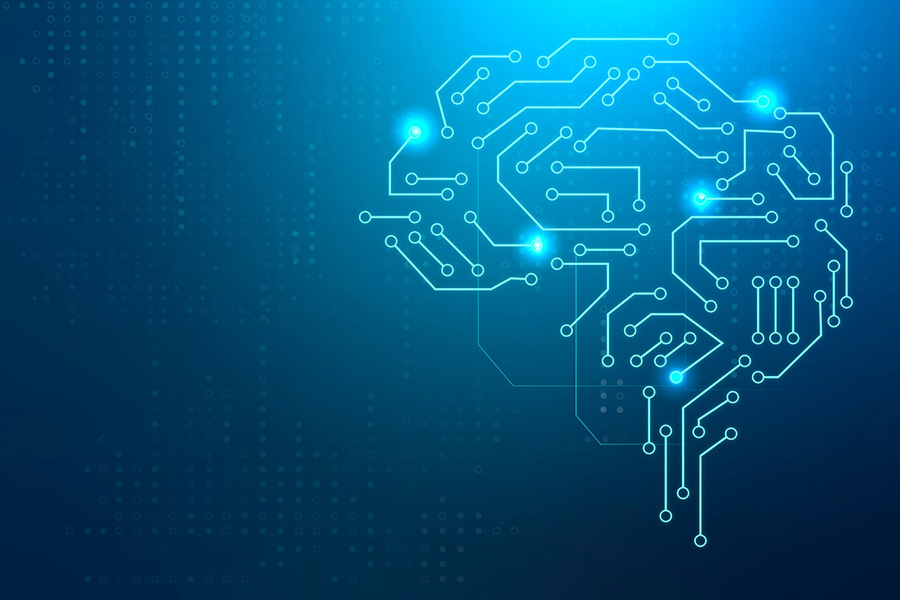
This chapter presents an overview of research on detecting hate speech in low-resource languages and explores application of various deep learning models for the task.
Authors
Deepawali Sharma, Department of Computer Science, Banaras Hindu University, Varanasi, Uttar Pradesh, India.
Vedika Gupta, Assistant Professor, Jindal Global Business School, O.P. Jindal Global University, Sonipat, Haryana, India.
Vivek Kumar Singh, Department of Computer Science, Banaras Hindu University, Varanasi, Uttar Pradesh, India.
Summary
During the recent years, online social media have expanded in volume and coverage and have become a significant source of information for different groups of people. The comments posted on social media can be emotion-laden and hence can create an impact on mental health of an individual or a group of individuals.
One such category of posts includes comments that are abusive or hateful in nature. The comments that spread hate and are abusive in nature usually target certain individuals or some specific communities. It is, therefore, very important to know about them and perhaps be able to detect such content in time. While there exist methods for automated detection of hate speech from posts in English language, there is relatively less research done on other low-resource languages, such as Tamil.
This chapter presents an overview of research on detecting hate speech in low-resource languages and explores application of various deep learning models for the task. The abusive comments are classified in different categories: Homophobia, Xenophobia, Transphobic, Misandry, Misogyny, Counter-speech, and Hope speech, from Tamil and Tamil–English code-mixed language. Those comments that are not in the Tamil language are categorized as “Not-Tamil.” The following deep learning models: recurrent neural network, long-short term memory (LSTM), and bidirectional LSTM, are applied to the task. Experimental results are presented along with an analysis of the quality of results.
Published in: Computational Intelligence Methods for Sentiment Analysis in Natural Language Processing Applications, Pages 207 – 226
To read the full chapter, please click here.