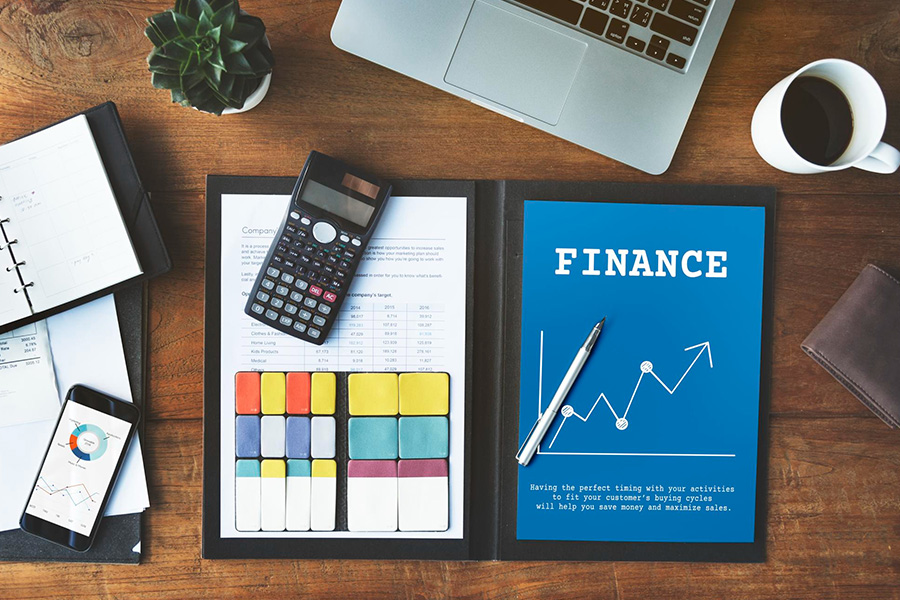
This is the first attempt to build network prediction models for cross market equity networks.
Authors
Biplab Bhattacharjee, Assistant Professor, Jindal Global Business School, O.P. Jindal Global University, Sonipat, Haryana, India.
Rajiv Kumar, Indian Institute of Management Kashipur, Kundeshwari, Kashipur, District- Udham Singh Nagar, Uttarakhand, India.
Arunachalam Senthilkumar, Amrita School of Business, Coimbatore, Amrita Vishwa Vidyapeetham, India.
Summary
Predicting financial networks has important implications in finance. However, less research attention has been given in this direction. This study aims to predict cross market linkage strengths in financial networks using dynamic edge weight prediction. The study builds edge weight prediction models using deep learning based unidirectional and bidirectional long short-term memory (LSTM and BiLSTM) approaches.
The models are built on temporally variant equity cross market networks in Asia. A rolling window approach is used to generate temporally synchronous observations of edge weight structures and subsequent networks. The models are trained with a series of historical network structure information. Tuning of hyperparameters was performed to obtain the optimized models.
The models were validated using nested cross validation methods. The applicability of the optimized models was assessed for different scenarios, and promising results were found for edge weight predictions on both unfiltered and filtered structures. The study also shows that the predictive performance of the BiLSTM model is the same across correlated (positively weighted) and anti-correlated (negatively weighted) edges.
However, for LSTM the same does not hold true. The results also demonstrate that the proposed models predict edge weights better during normal conditions than in the crisis period. The proposed models are benchmarked against ARIMA and RNN. To our knowledge, this is the first attempt to build network prediction models for cross market equity networks. The findings can have key implications such as managing international portfolio diversifications and controlling systemic risk transmissions.
Published in: International Review of Financial Analysis
To read the full article, please click here.