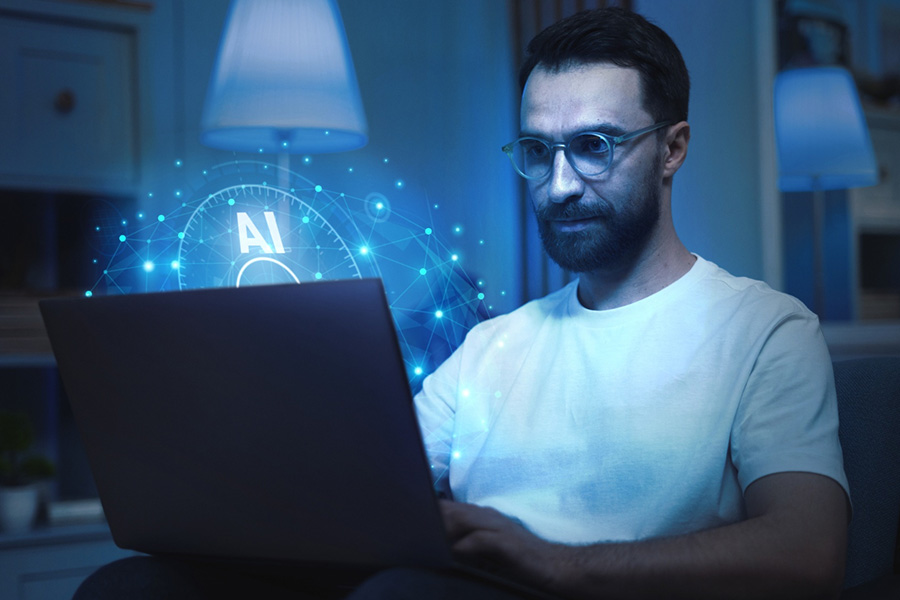
The study demonstrates the feasibility of AI models for predicting delays in Indian courts, based on relevant data points such as jurisdiction, court, judge, subject, and the parties involved.
Authors
Mohit Bhatnagar, Associate Professor, Jindal Global Business School, O.P. Jindal Global University, Sonipat, Haryana, India.
Shivaraj Huchhanavar, Assistant Professor, Jindal Global Law School, O.P. Jindal Global University, Sonipat, Haryana, India.
Summary
This paper presents a classification model that predicts delays in Indian lower courts based on case information available at filing. The model is built on a dataset of 4.2 million court cases filed in 2010 and their outcomes over a 10-year period. The data set is drawn from 7000+ lower courts in India.
The authors employed AutoML to develop a multi-class classification model over all periods of pendency and then used binary decision forest classifiers to improve predictive accuracy for the classification of delays. The best model achieved an accuracy of 81.4%, and the precision, recall, and F1 were found to be 0.81.
The study demonstrates the feasibility of AI models for predicting delays in Indian courts, based on relevant data points such as jurisdiction, court, judge, subject, and the parties involved.
The paper also discusses the results in light of relevant literature and suggests areas for improvement and future research. The authors have made the dataset and Python code files used for the analysis available for further research in the crucial and contemporary field of Indian judicial reform.
Published in: García Márquez, F.P., Jamil, A., Ramirez, I.S., Eken, S., Hameed, A.A. (eds) Computing, Internet of Things and Data Analytics. ICCIDA 2023. Studies in Computational Intelligence, vol 1145. Springer, Cham.
To read the full paper, please click here.