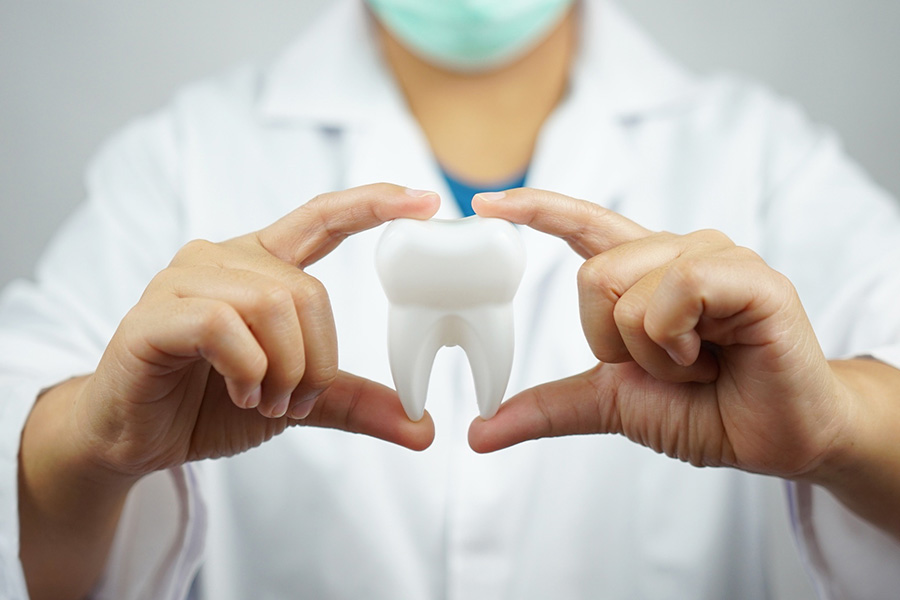
In this study, the researchers propose a pipelined Deep Neural Network (DNN) approach to detect healthy and non-healthy periapical dental X-ray images.
Authors
Vedika Gupta, Assistant Professor, Jindal Global Business School, O.P. Jindal Global University, Sonipat, Haryana, India.
Dipit Vasdev, Department of Computer Science and Engineering, Bharati Vidyapeeth’s College of Engineering, New Delhi, India.
Shubham Shubham, Department of Computer Science and Engineering, Bharati Vidyapeeth’s College of Engineering, New Delhi, India.
Ankit Chaudhary, Department of Computer Science and Engineering, Bharati Vidyapeeth’s College of Engineering, New Delhi, India.
Nikita Jain, Department of Computer Science and Engineering, Bharati Vidyapeeth’s College of Engineering, New Delhi, India.
Mehdi Salimi, Department of Mathematics and Statistics, St. Francis Xavier University, Antigonish, NS, Canada; Center for Dynamics, Faculty of Mathematics, Technische Universität Dresden, Dresden, Germany.
Ali Ahmadian, Department of Law, Economics and Human Sciences and Decisions Lab, Mediterranea University of Reggio Calabria, 89125, Reggio Calabria, Italy; Department of Mathematics, Near East University, Nicosia, TRNC, Mersin 10, Turkey
Summary
This paper studies the problem of detection of dental diseases. Dental problems affect the vast majority of the world’s population. Caries, RCT (Root Canal Treatment), Abscess, Bone Loss, and missing teeth are some of the most common dental conditions that affect people of all ages all over the world. Delayed or incorrect diagnosis may result in mistreatment, affecting not only an individual’s oral health but also his or her overall health, thereby making it an important research area in medicine and engineering.
We propose a pipelined Deep Neural Network (DNN) approach to detect healthy and non-healthy periapical dental X-ray images. Even a minor enhancement or improvement in existing techniques can go a long way in providing significant health benefits in the medical field.
This paper has made a successful attempt to contribute a different type of pipelined approach using AlexNet in this regard. The approach is trained on a large dataset of 16,000 dental X-ray images, correctly identifying healthy and non-healthy X-ray images. We use an optimized Convolutional Neural Networks and three state-of-the-art DNN models, namely Res-Net-18, ResNet-34, and AlexNet for disease classification.
In our study, the AlexNet model outperforms the other models with an accuracy of 0.852. The precision, recall and F1 scores of AlexNet also surpass the other models with a score of 0.850 across all metrics. The area under ROC curve also signifies that both the false-positive rate and false-negative rate are low. We conclude that even with a big data set and raw X-ray pictures, the AlexNet model generalizes effectively to previously unseen data and can aid in the diagnosis of a variety of dental diseases.
Published in: Annals of Operations Research
To read the full article, please click here.