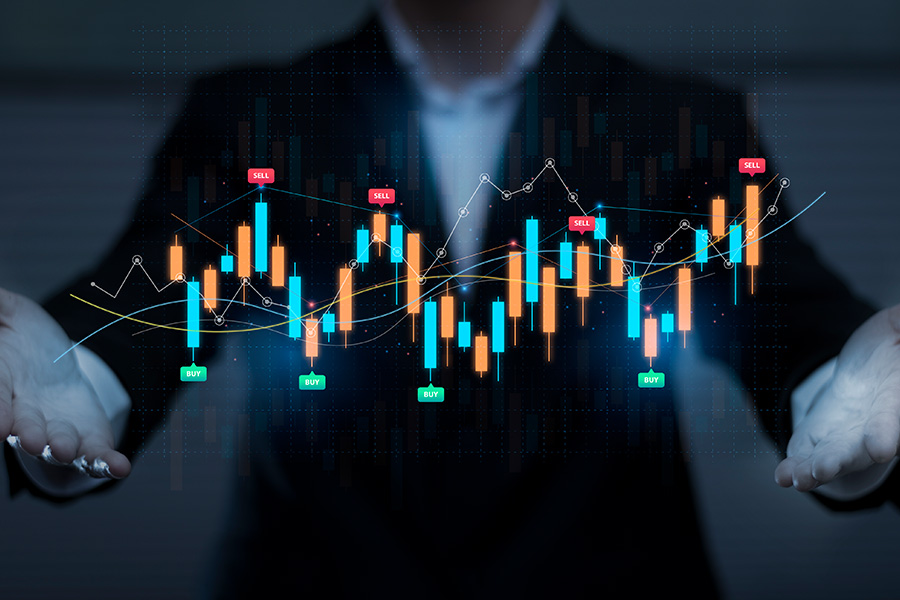
Rule-based trading concentrates on utilizing technical analysis tools to generate buy and sell signals, aiming for profitability.
Authors
Sarveshwar Kumar Inani, Assistant Professor, Jindal Global Business School, O.P. Jindal, Global University, Sonipat, Haryana, India.
Harsh Pradhan, Faculty of Management Studies, Banaras Hindu University, India.
Surender Kumar, Jaipuria Institute of Management, Noida Campus, India.
Baidyanath Biswas, Trinity Business School, Trinity College, Business Analytics Area, Dublin, Ireland.
Summary
In stock markets, technical analysis plays a vital role by offering valuable insights into price trends, patterns, and anticipated market movements, aiding investors in making well-informed decisions. This study employs bibliometric and topic modelling approaches on 589 English-language journal articles indexed in Scopus in the last two decades (from 2003 to 2023), exclusively focusing on technical analysis in stock markets. The keyword co-occurrence analysis identifies five topic clusters.
The application of structural topic modelling also unravels five prominent thematic clusters, namely pattern-based forecasting, rule-based trading, algorithmic trading, techno-fundamental trading, and machine learning & sentiment analysis. The topic of pattern-based forecasting involves researching the application of various patterns or models to predict stock prices.
Rule-based trading concentrates on utilizing technical analysis tools to generate buy and sell signals, aiming for profitability. The algorithmic trading cluster explores the use of algorithms to systematically execute buy and sell actions, especially in high-frequency trading scenarios. Techno-fundamental trading investigates the integration of both fundamental and technical analysis in trading and investment decisions.
Lastly, machine learning & sentiment analysis focus on applying advanced machine learning techniques and sentiment analysis for predicting stock prices, highlighting the use of sophisticated methods in this domain. The three predominant topics in the dataset are “rule-based trading,” “machine learning & sentiment analysis,” and “algorithmic trading” constituting 26.79%, 23.52%, and 21.11% of the dataset, respectively. These findings underscore the prominence and significance of these themes within the context of the research domain.
Published in: Investment Management and Financial Innovations
To read the full article, please click here.