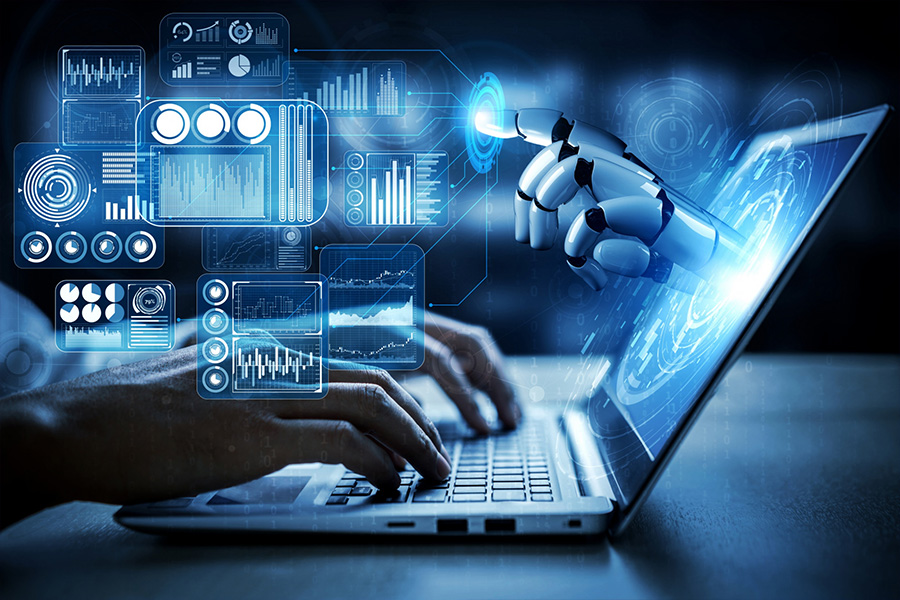
In this paper, the researchers use the Apriori algorithm in conjunction with other machine learning tools to cluster the potential backers and provide more accurate recommendations for crowdfunding projects.
Authors
Sachin Kumar Mangla, Full Professor and Director, Research Center for Digital Circular Economy for Sustainable Development Goals (DCE-SDG), Jindal Global Business School, O.P. Jindal Global University, Haryana, India.
Shaojian Qu, School of Management Science and Engineering, Nanjing University of Information Science and Technology, Nanjing, People’s Republic of China.
Lei Xu, Economics and Management College, Civil Aviation University of China, Tianjin, People’s Republic of China.
Felix T. S. Chan, Department of Decision Sciences, Macau University of Science and Technology, Taipa, Macao.
Jianli Zhu, School of Management, University of Shanghai for Science and Technology, Shanghai, People’s Republic of China.
Sobhan Arisian, La Trobe Business School, La Trobe University, Melbourne, Australia.
Summary
Traditional clustering methods fail to accurately cluster the feature vectors of backers and match the potential backers to compatible crowdfunding projects, mainly due to their sensitivity to the setting of the initial value. In this paper, we use the Apriori algorithm in conjunction with other machine learning tools to cluster the potential backers and provide more accurate recommendations for crowdfunding projects.
Focusing on potential projects listed in a major reward-based crowdfunding platform, we first train the data obtained from the available list of backers. Using the Apriori algorithm, the degree of association between different project backers is then obtained, and weight calculation of the backers is carried out according to the association degree of the backers.
The degree of association is used as a key index to cluster similar backers. Finally, we test the model and determine whether clustering can correctly classify the data in the test set based on the Apriori algorithm. Our experimental results show that there is 90% accuracy, precision and recall of the model. The proposed solution outperforms the other five benchmark methods and offers an imporved matchmaking by connecting the listed crowdfunding projects to the right backers.
Published in: International Journal of Production Research
To read the full article, please click here.