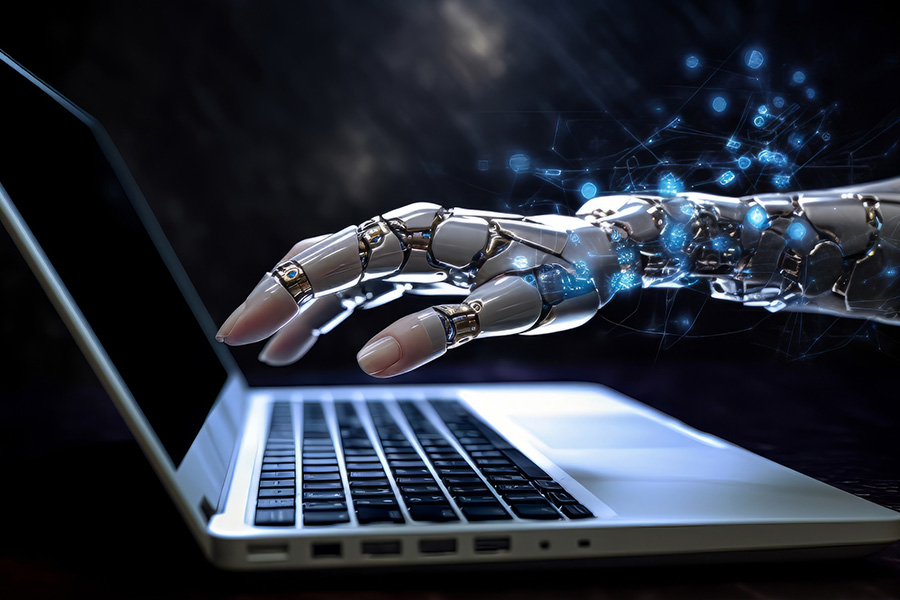
By applying machine learning techniques and combining Lexicon-based methods with machine learning algorithms, this study aims to enhance sentiment classification for better customer relationship management and product development.
Authors
Ritanjali Panigrahi, Assistant Professor, Jindal Global Business School, O.P. Jindal Global University, Sonipat, Haryana, India.
Nishikant Bele, Department of Digital Health, International Institute of Health Management Research, New Delhi, India.
Prabin Kumar Panigrahi, Indian Institute of Management Indore, Prabandh Shikhar, Indore, Madhya Pradesh, India.
Brij B. Gupta, Department of Computer Science and Information Engineering, Asia University, Taichung 413, Taiwan, and Kyung Hee University, 26 Kyungheedae-ro, Dongdaemun-gu, Seoul 02447, Korea, and Symbiosis Centre for Information Technology (SCIT), Symbiosis International University, Pune, India, and Center for Interdisciplinary Research, University of Petroleum and Energy Studies (UPES), Dehradun, India
Summary
This study explores feature-level sentiment analysis of Hindi blog reviews in enterprise systems, a significant area in the Indian context yet understudied.
By applying machine learning techniques like SVM across unigram, bigram, trigram, and n-gram models, and combining Lexicon-based methods with machine learning algorithms, we aim to enhance sentiment classification for better customer relationship management and product development.
Contrasting with document-level approaches, our method focusing on bigrams achieves a test accuracy of 75%, offering a scalable model for enterprises to extract detailed customer insights from informal text, thereby aiding informed decision-making in a multicultural environment.
Published in: Enterprise Information Systems
To read the full article, please click here.