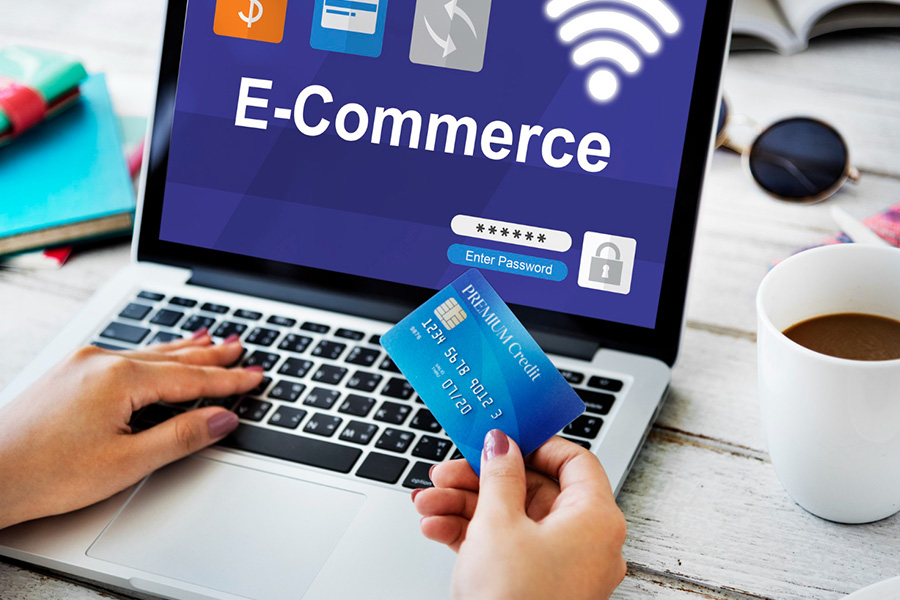
This study explores ways to predict product returns in e-commerce by comparing different models. It found that the Bayesian-optimized XGBoost model performed the best, with over 77% accuracy.
Authors
Biplab Bhattacharjee, Associate Professor, Jindal Global Business School, O.P. Jindal Global University, Sonipat, Haryana, India and Information Systems and Analytics Area, Indian Institute of Management, Shillong, India
Kavya Unni, Department of Management, Amrita Vishwa Vidyapeetham, Amritapuri Campus, Amritapuri, India
Maheshwar Pratap, Department of Management, Amrita Vishwa Vidyapeetham, Amritapuri Campus, Amritapuri, India
Summary
Product returns are a major challenge for e-businesses as they involve huge logistical and operational costs. Therefore, it becomes crucial to predict returns in advance. This study aims to evaluate different genres of classifiers for product return chance prediction, and further optimizes the best performing model.
Methodology
An e-commerce data set having categorical type attributes has been used for this study. Feature selection based on chi-square provides a selective features-set which is used as inputs for model building. Predictive models are attempted using individual classifiers, ensemble models and deep neural networks. For performance evaluation, 75:25 train/test split and 10-fold cross-validation strategies are used. To improve the predictability of the best performing classifier, hyperparameter tuning is performed using different optimization methods such as, random search, grid search, Bayesian approach and evolutionary models (genetic algorithm, differential evolution and particle swarm optimization).
Findings
A comparison of F1-scores revealed that the Bayesian approach outperformed all other optimization approaches in terms of accuracy. The predictability of the Bayesian-optimized model is further compared with that of other classifiers using experimental analysis. The Bayesian-optimized XGBoost model possessed superior performance, with accuracies of 77.80% and 70.35% for holdout and 10-fold cross-validation methods, respectively.
Research Limitations
Given the anonymized data, the effects of individual attributes on outcomes could not be investigated in detail. The Bayesian-optimized predictive model may be used in decision support systems, enabling real-time prediction of returns and the implementation of preventive measures.
Originality/value
There are very few reported studies on predicting the chance of order return in e-businesses. To the best of the authors’ knowledge, this study is the first to compare different optimization methods and classifiers, demonstrating the superiority of the Bayesian-optimized XGBoost classification model for returns prediction.
Published in: Journal of Systems and Information Technology
To read the full article, please click here.