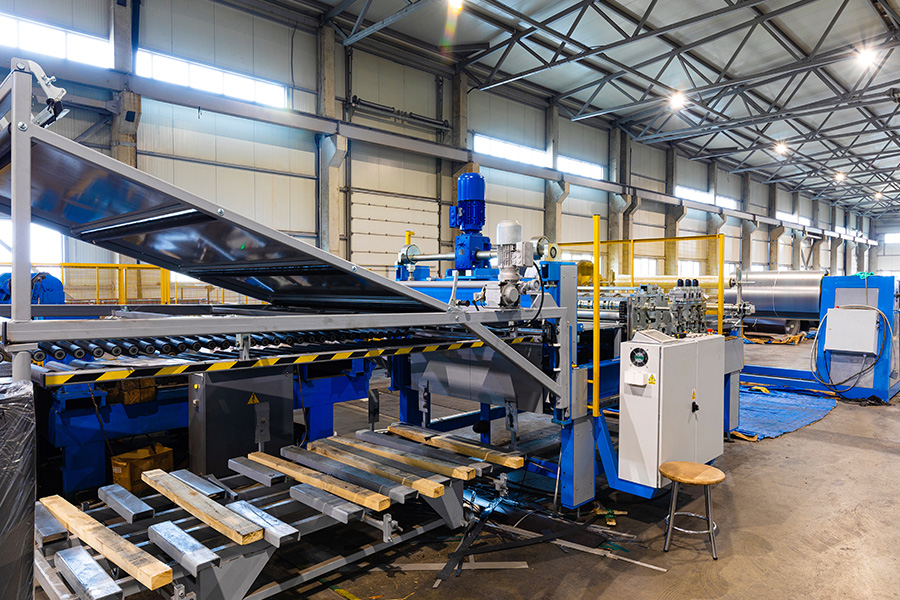
In this study, the researchers propose a model, Clustering Based Classifier Ensemble Method for Cost of Defect Prediction (CBCEM-CoD), incorporating clustering, classification, prediction, and learning techniques of Big Data Analytics for quality management in the manufacturing industry.
Authors
Sachin Kumar Mangla, Full Professor, and Director, Research Centre ‘Digital Circular Economy for Sustainbale Development Goals (DCE-SDG), Jindal Global Business School, O P Jindal Global University, Haryana, India.
Gorkem Sariyer, Yasar, University, Department of Business Administration, Izmir, Turkey.
Yigit Kazancoglu, Yasar University, Department of Logistics Management, Izmir, Turkey.
Lei Xu, Professor of Economics and Management College, Civil Aviation University of China, Tianjin, China.
Ceren Ocal Tasar, Department of Computer Engineering, Yasar University, Turkey.
Summary
Due to technological advances, Big Data Analytics (BDA) has become increasingly important over the last few years. This has led companies to evolve BDA capabilities (BDAC) to manage operations and make better decisions.
In this study, we propose a model, Clustering Based Classifier Ensemble Method for Cost of Defect Prediction (CBCEM-CoD), incorporating clustering, classification, prediction, and learning techniques of BDA for quality management in the manufacturing industry.
CBCEM-CoD (1) is fact-driven, as it is based on a fundamental problem of the manufacturing industry, (2) integrates different BDA techniques in a specific way when an output of one technique is used as an input of another, and (3) extracts insights from real-world big data and directly offers many implications for practice.
In the first stage of the CBCEM-CoD, k-means and agglomerative clustering techniques are used comparatively for segmenting customers and products. CoD values of each product and customer segment are predicted using ensemble learning techniques in the second stage. The model is tested using a case data set from the kitchenware industry.
As a result, 53 and 720 different types of customers and products in the train data set are segmented in optimal numbers of 4 and 20 clusters. Around 89% accuracy is obtained for CoD predictions in the test data set.
These results have substantial business value since they inform managers how to prioritize their focus on specific products and customer types to reduce the cost of a defect. We also highlight the importance of developing BDAC in dynamically changing environments to create a competitive advantage.
Published in: Computers & Industrial Engineering
To read the full article, please click here.