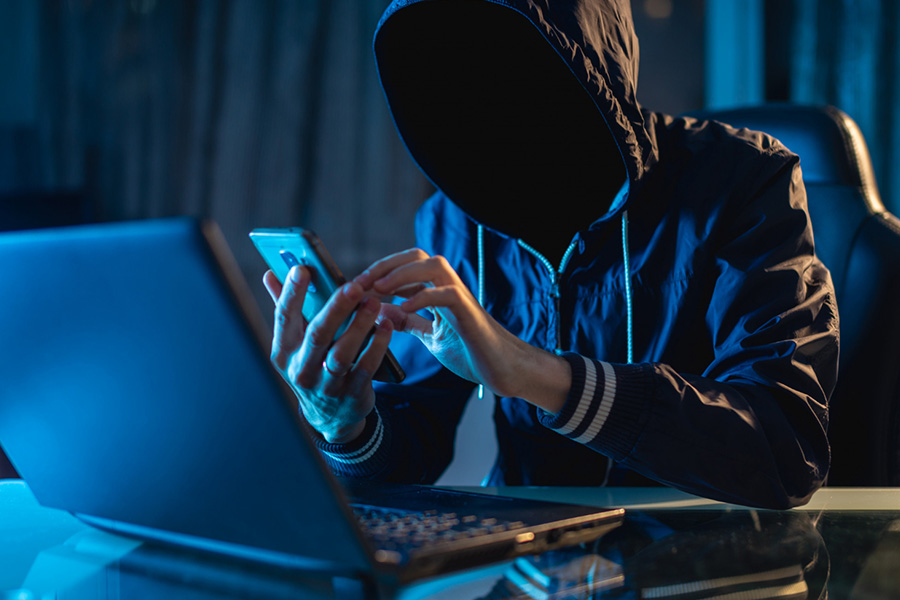
Machine learning algorithms effectively predict crime rates, providing valuable insights for crime prevention and reduction.
Authors
Pranav Dass, Department of Computer Science, Shyam Lal College, University of Delhi, Delhi, 110032, India
Vedika Gupta, Associate Professor, Jindal Global Business School, O.P. Jindal Global University, Sonipat, Haryana, India
Shreya Dhingra, Department of Computer Science Engineering, Bharati Vidyapeeth’s College of Engineering, New Delhi, 110063, India
Rohan Arora, Department of Computer Science Engineering, Bharati Vidyapeeth’s College of Engineering, New Delhi, 110063, India
Piyush Katariya, Department of Computer Science Engineering, Bharati Vidyapeeth’s College of Engineering, New Delhi, 110063, India
Adarsh Kumar, Department of Computer Science Engineering, Bharati Vidyapeeth’s College of Engineering, New Delhi, 110063, India
Summary
Crime is a detrimental socioeconomic issue that impacts individuals globally. Crime significantly affects a nation’s standard of living, financial well-being, and standing. Over the past few years, there has been a significant increase in the crime rate. Law enforcement must implement proactive measures to mitigate crime rates. Enhanced technologies and innovative methods are required to bolster crime analytics and safeguard communities. Precise and up-to-date crime predictions can reduce crime rates, but they provide a complex problem for scientists due to the multiple factors that drive crime occurrences.
This study employs a range of visualisation techniques and machine learning algorithms to predict the spread of crime across a vast geographical area. The datasets were evaluated and presented in the initial phase, based on their relevance. Subsequently, machine learning algorithms were employed to extract insights from extensive datasets and uncover concealed correlations within the data. This information was subsequently utilised to identify and analyse crime patterns, providing crime analysts with valuable tools for crime prediction.
Consequently, this approach proves advantageous in the realm of crime prevention. Every day, a substantial number of criminal activities are committed. The information in this instance includes both the date and the crime rate for the relevant years. The crime rate in this project is determined by employing various distinct criminal classifications. Utilising historical data, we employ the RNN, LSTM, and GRU algorithms to forecast the future percentage of the crime rate. The programme receives the date as an input and produces the crime rate percentage for that specific year.
Published in: Journal of Discrete Mathematical Sciences and Cryptography
To read the full article, please click here.