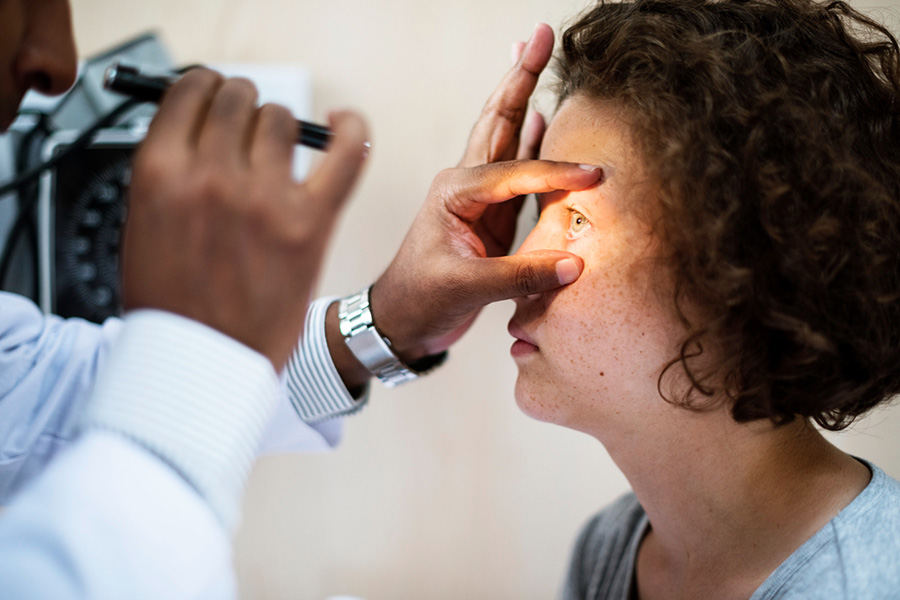
A hybrid method combining statistical-textural and intensity features improves retinal disease detection using machine learning classifiers, showing promising results for early intervention.
Authors
D Kalyani, Department of Information Technology, Vallurupalli Nageswara Rao Vignana Jyothi Institute of Engineering and Technology, India
Jakkala Ravichandar Reddy, CSE, Vijay Rural Engineering College, India
Cindhe Ramesh, Department of Computer Science and Engineering, CVR College of Engineering, Vastunagar, Mangalpally(v), Telangana, Ibrahimpatnam, 501510, India
Sowmya Saraf, Department of Computer Science and Engineering, Mallareddy University, India
Ashish Kaushal, Assistant Professor, Jindal Global Business School, O.P. Jindal Global University, Sonipat, Haryana, India
Jihane Ben Slimane, Department of Computer Sciences, Faculty of Computing and Information Technology, Northern Border University, Rafha, 91911, Saudi Arabia
Albia Maqbool, Department of Computer Sciences, Faculty of Computing and Information Technology, Northern Border University, Rafha, 91911, Saudi Arabia
Summary
Retinal imaging features such as fundus lesions are crucial signs of several ocular diseases, such as diabetic retinopathy and age-related macular degeneration. The early and accurate detection of the aforementioned abnormalities is essential for correct diagnosis and therapeutic management. In this study, we propose a new hybrid statistical-textural and intensity feature-based method for the improvement of the discrimination between retinal diseases with machine learning classifiers.
By incorporating statistical texture analysis to measure changes in pixel intensity, this method not only extracts features based on intensities of fundus images but also serves as the comparison tool for a complete evaluation of the retina. A robust feature set is developed by merging intensity-based features with several texture descriptors including GLCM, LBP etc. We extract these features and then use them to train and test the performance of a number of classifiers, such as Support Vector Machines (SVM) and Random Forests for more accurate detection.
Experiments have been conducted on a public dataset of retinal images and with the support vector machine classifier, encouraging results compared to purely texture-based or intensity-based methods in terms of classification accuracy are obtained. The overall findings of this study underscore the promise of integrating both statistical-textural and intensity features for improved retinal disease detection process, which will serve as an important stepping stone for future investigations and production of automated screening tools in retinal. The method also holds potential to enable early intervention in the development of vision-related diseases and, ultimately, decrease their impact.
Published in: Journal of Information Systems Engineering and Management
To read the full article, please click here.