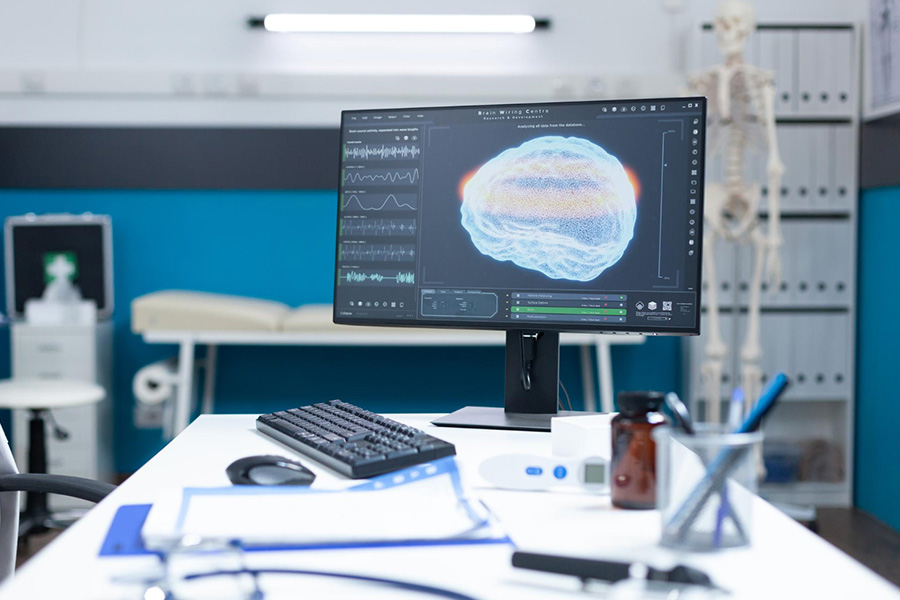
SWCNet, a novel CNN-based model, achieves state-of-the-art accuracy (up to 97.42%) in classifying motor imagery EEG signals, demonstrating its potential to enhance BCI applications in medical rehabilitation.
Authors
Kamal Singh, National Institute of Technology (NIT) Delhi, ECE Department, Delhi, 110036, India
Nitin Singha, National Institute of Technology (NIT) Delhi, ECE Department, Delhi, 110036, India
Gaurav Jaswal, Indian Institute of Technology, Kamand, Himachal Pradesh, Mandi, 175005, India
Swati Bhalaik, Assistant Professor of Practice, Jindal Global Business School, O.P. Jindal Global University, Haryana, Sonipat, Haryana, India
Summary
The major challenge in fully utilizing the motor imagery-based brain-computer interface (MI-BCI) capabilities is accurately classifying the MI electroencephalography (MI-EEG) signals. Despite numerous advancements in signal processing and deep learning techniques, there is significant scope for improvement in the accuracy currently available in the state-of-the-art. This can be achieved by leveraging spatial and temporal features of MI-EEG signal.
We propose SWCNet, a convolutional neural network (CNN)-based model, and integrate it with the sliding window technique to increase the accuracy. In this work, a new CNN architecture has been proposed to extract more features from data, whereas the sliding window technique enhances temporal features by augmenting the input sensor data along the temporal dimension. We have thoroughly evaluated the performance of SWCNet using subject-dependent and subject-independent approaches for four different datasets.
Our analysis includes general accuracy metrics, an ablation study, a parametric sensitivity study, and a detailed class-wise performance evaluation for the tongue, foot, left-hand, and right-hand movements. The proposed model achieves accuracies of 97.42%, 94.46%, 92.27%, and 90.82% for BCI Competition IV-2a, BCI Competition IV-2b, High Gamma, and OpenBMI datasets, respectively. SWCNet outperforms the state-of-the-art methods with higher accuracy for all the datasets, demonstrating its superior generalizability. SWCNet holds promise in enhancing the effectiveness of BCI applications, especially in medical rehabilitation.
Published in: IEEE Sensors Journal
To read the full article, please click here.